Smart wearable devices in cardiovascular care: where we are and how to move forward
Technological innovations reach deeply into our daily lives and an emerging trend supports the use of commercial smart wearable devices to manage health. In the era of remote, decentralized and increasingly personalized patient care, catalysed by the COVID-19 pandemic, the cardiovascular community must familiarize itself with the wearable technologies on the market and their wide range of clinical applications. In this Review, we highlight the basic engineering principles of common wearable sensors and where they can be error-prone. We also examine the role of these devices in the remote screening and diagnosis of common cardiovascular diseases, such as arrhythmias, and in the management of patients with established cardiovascular conditions, for example, heart failure. To date, challenges such as device accuracy, clinical validity, a lack of standardized regulatory policies and concerns for patient privacy are still hindering the widespread adoption of smart wearable technologies in clinical practice. We present several recommendations to navigate these challenges and propose a simple and practical ‘ABCD’ guide for clinicians, personalized to their specific practice needs, to accelerate the integration of these devices into the clinical workflow for optimal patient care.
Key points
- Smart wearables generate a plethora of data through various sensors and software algorithms and understanding their basic engineering principles and limitations can be helpful for clinicians and scientists.
- Evidence supports the use of wearable devices in cardiovascular risk assessment and cardiovascular disease prevention, diagnosis and management, but large, well-designed trials are needed to establish their advantages.
- Several challenges still hinder the widespread adoption of wearables in clinical practice, including a concern for device accuracy, patient privacy and cost, and how to separate actionable data from noise.
- Overcoming these challenges requires that various stakeholders come together to develop comprehensive evaluation frameworks, pragmatic regulatory policies, clinical trials and medical education curricula.
- A practical ‘ABCD’ guide for clinicians can facilitate the integration of these devices in routine clinical practice.
Similar content being viewed by others
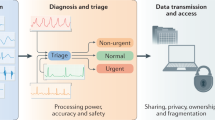
Integration of novel monitoring devices with machine learning technology for scalable cardiovascular management
Article 09 October 2020
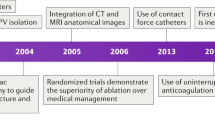
Looking back and thinking forwards — 15 years of cardiology and cardiovascular research
Article 30 September 2019
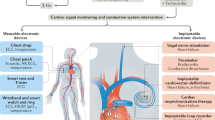
Self-powered cardiovascular electronic devices and systems
Article 07 September 2020
Introduction
Technological innovations continue to become exceedingly ingrained into everyday life and consumers are beginning to use consumer-grade software and hardware devices to manage their health. Smart wearables are consumer-grade, connected electronic devices that can be worn on the body as an accessory or embedded into clothing. These include smartwatches, rings and wristbands, to name a few, and they all have high processing power and numerous sophisticated sensors that can glean new health insights. An estimated 20% of US residents currently own a smart wearable device and the global market is expected to grow at a compound annual growth rate of 25%, reaching US$70 billion by 2025 (refs 1,2 ). Although the integration of this technology in the clinical workplace is still in its infancy, it has rapidly moved through the Gartner Hype Cycle for emerging technologies 3 and its adoption has further accelerated after the coronavirus disease 2019 (COVID-19) pandemic and the explosive growth of telehealth 4 . In this Review, we summarize the basic engineering principles of common wearable sensors and discuss their applications in cardiovascular disease prevention, diagnosis and management. We also highlight several challenges hindering their widespread adoption and how to move forward as we embark on a new decade of clinical innovation. Finally, we propose a practical ‘ABCD’ guide for clinicians to handle wearables in routine clinical practice.
Engineering principles of wearable sensors
Activity sensors
Physical activity is inversely correlated to adverse cardiovascular outcomes 5 and all-cause mortality and is recommended by the AHA as one of the ‘Life’s Simple 7’ lifestyle recommendations to promote heart health 6 . The assessment of physical activity levels has traditionally been subjective and recorded only during clinic visits, if at all. This approach is limited by a lack of sufficient detail, recall bias and a failure to objectively assess physical activity in a real-life environment. Common statements such as “I walk five times a week for 30 minutes” do not include important information such as physical activity intensity, distance and sedentary time. Therefore, the subjective reporting of physical activity levels will become obsolete as digital health trends, such as wearables and smartphones, can objectively and accurately assess physical activity and energy expenditure through various sensors.
The triaxial accelerometer is the dominant method of activity monitoring in current wearables and measures linear acceleration along three different planes. The other major inertial sensor is the gyroscope, which measures angular motion 7 . The common operation principle of triaxial accelerometers is based on a seismic mass attached to a mechanical suspension system 7 . These devices take advantage of Newton’s second law in which mass deflection to the opposite direction of motion with a certain amount of acceleration can be measured electrically. The three most common types of accelerometers are the piezoresistive, piezoelectric and differential capacitive accelerometers, with the differential capacitive accelerometer being the most commonly used in wearables owing to its superior performance 7 . In differential capacitive accelerometers, the seismic mass is suspended between two electrodes and the acceleration of the seismic mass is proportional to the differential capacitance between the electrodes 7 . The advantages of these accelerometers include low power consumption, fast response to motion and superior accuracy compared with the piezoresistive model, which can be undermined by its temperature sensitivity 7 . The placement site on the human body is one of the most important factors that affect the accuracy of accelerometer measurements 7 . A centrally located sensor on the torso (embedded into vests, mounted by straps or attached to the skin) is best suited for detecting posture, acceleration and whole-body movements and offers the least errors compared with other body locations 7 . An ankle-mounted sensor is better suited to measure steps and energy expenditure. However, wrist placement has taken precedence over ankle placement in commercial wearables owing to increased compliance and convenience 8 .
Global Positioning System (GPS) and barometers are also included in wearables to more accurately assess physical activity. GPS utilizes a system of 24 or more satellites that are continuously emitting signals to identify their precise orbital position and time based on extremely accurate and stable atomic clocks 9 . With the use of complex equations that include signal emission time and speed of light and account for Einstein’s relativistic concepts, the GPS receiver can determine its distance from at least four satellites. The receiver can then trilaterate its position on earth with an accuracy of up to 4.9 m (ref. 9 ). However, GPS function is limited by satellite geometry, signal blockage, building reflections, atmospheric conditions and receiver design features 9 . By contrast, barometers utilize a diaphragm mounted on a vacuum chamber that compresses proportional to pressure. The amount of deformation of the diaphragm is converted to electrical signals via a capacitive model that relies either on plates moving closer together or on a piezoresistive strain model in which resistors on the diaphragm change their electrical resistance according to the extent of deformation 10 . The barometer is then able to determine changes in altitude, track stair count and detect falls on the basis of the principle that atmospheric pressure decreases with increasing altitude 10 . However, barometric measurements can be error-prone because the device might mistake natural changes in ambient temperature and pressure as a change in altitude 10 . Although the use of more sensors leads to better estimation of physical activity and energy expenditure, this approach can also put further strain on battery life 11 .
Heart rate and rhythm sensors
Heart rate (HR) measurements during rest and exercise can be used to predict the risk of cardiovascular disease. In healthy populations, a high resting HR has been associated with an increased risk of coronary artery disease and all-cause death 12 and is also well recognized as a predictor of adverse outcomes in patients with heart failure (HF) 13 . An impaired HR recovery after exercise correlates with increased adverse cardiovascular events 14 . HR variability (HRV) has also been strongly linked to the risk of adverse cardiovascular events in healthy individuals and in patients with HF with reduced ejection fraction 15 .
Commercial wearables measure HR and heart rhythm through electrocardiography (ECG) or photoplethysmography (PPG) by calculating beat-to-beat time intervals and using algorithms to classify heart rhythm. ECG sensors come in various forms and are the gold standard for HR and heart rhythm measurement. Chest-strap monitors and ECG patches provide continuous monitoring of heart rhythm but are less appealing to the average consumer than other options such as smartwatches given their bulkiness, limited functions and long-term inconvenience. Some smartwatches can record a single-lead ECG as needed by placing a contralateral finger on the crown (negative electrode on the side of the watch), with the back of the watch serving as the positive electrode 16 . Single-lead ECGs are useful to diagnose simple and common arrhythmias such as atrial fibrillation (AF). However, these single-lead ECGs are often insufficient for the accurate diagnosis of more complex arrhythmias and other conditions such as myocardial infarction (MI) or to detect interval abnormalities unless specific manoeuvres are deployed 17 .
PPG measures changes in microvascular blood volume that translate into pulse waves and a tachogram recording 18 . An emitter sends a continuous pulse of photons through the skin and a photodetector measures the variable intensity of reflected photons from the tissue 18 . Most wearables continuously activate the PPG during exercise whereas, during rest and sleep, PPG measurements occur only intermittently to preserve battery life. PPG tachograms, especially when augmented by single-lead ECG, can also identify arrhythmias 19 . Nevertheless, PPG technology has limitations. The main drawback is that the sensor works best when in direct contact with the skin, which is not always the case with wearables secured with straps. Skin colour, moisture and even tattoos have also been postulated to affect PPG accuracy 20 , although one study showed similar device performance across a full range of skin tones 21 .
Given the variability in HR accuracy of PPG sensors across different wearable devices, a number of studies have directly compared their performance. One study that investigated the accuracy of the Apple Watch 3 (Apple, USA) and the Fitbit Charge 2 (Fitbit, USA) showed that both devices provided an acceptable PPG sensor HR accuracy (rc = 0.99); the accuracy of wrist-worn devices varied substantially by the type of activity and 5% of HR measurements were substantially inaccurate 23 . These studies highlight that PPG HR readings from wrist-worn devices during activity should be interpreted with caution 20 , whereas less convenient, chest-strap monitors are more accurate. Further studies examining newer devices and their performance in diverse populations are needed to further understand the limits of PPG technology and improve its performance.
Blood pressure sensors
Hypertension is a leading cause of morbidity and mortality globally 24 . Incorporating accurate blood pressure (BP) measurement within consumer-grade wearables has the potential to improve screening for hypertension and identify nocturnal or exercise hypertension, which have been linked to worse outcomes 24 . The HeartGuide wristwatch (Omron, Japan), with a built-in cuff, was compared with an ambulatory BP device in office and ambulatory conditions 25 . For office BP measurements, patients were seated and wearing the HeartGuide wristwatch and the standard BP measurement device in the same non-dominant arm and BP readings were taken twice by each device in alternating 30–60-second intervals. For ambulatory BP measurements, patients were given an ambulatory, upper-arm machine that measures BP at 30-minute intervals over 24 h and were instructed to use the HeartGuide device after each ambulatory BP measurement at least 10 times while awake. The mean difference (±s.d.) in systolic BP between both groups was 0.8 ± 12.8 mmHg in the office-based setting and 3.2 ± 17.0 mmHg in the ambulatory setting 25 . These findings are consistent with previously described limitations of wrist-based cuff BP measurements 26 . BP can now be measured without a cuff, increasing the feasibility and ease of monitoring BP throughout the day. This technology uses a combination of PPG and ECG measurements to estimate BP by calculating the pulse transit time, that is, the time required for the arterial pressure wave to travel from the heart to a distant vessel 27 . In a small study, cuff-less BP measurements were compared with ambulatory device measurements 28 . Mean biases between the wearable and ambulatory devices over 24 h were 0.5 mmHg (–10.1 mmHg to 11.1 mmHg) for systolic BP and 2.24 mmHg (–17.6 mmHg to 13.1 mmHg) for diastolic BP, with the mean bias widening over 7 days to –12.7 mmHg for systolic BP and –5.6 mmHg for diastolic BP. Several other variables have been considered to measure cuff-less BP such as pulse wave velocity and propagation in combination with deep learning algorithms; however, most of the studies are limited by small sample sizes and a lack of external validation 29 . Although encouraging, cuff-less BP monitoring is still in its infancy and requires further scrutiny.
Other sensors
Biochemical sensors can measure body fluid electrolytes with the use of electrochemical transducers, offering valuable information about plasma volume status and analyte concentrations 30 . However, the accuracy of these sensors changes with skin temperature, skin contamination with dust, dried sweat or other substances, and hair density. One example of biochemical sensors are the minimally invasive continuous glucose monitors that have been clinically validated but are difficult to embed in consumer-grade wearables and mostly function as a stand-alone product 31 . Non-invasive sensors of sweat and saliva might be more practical to integrate into wearables but still need to be carefully evaluated 32 .
Biomechanical sensors incorporated into clothing or shoes, such as ballistocardiograms, seismocardiograms and dielectric sensors, have been developed in an attempt to passively and continuously measure variables such as cardiac output, lung fluid volume and weight 1 , which could be beneficial in managing conditions such as HF. Other biomechanical sensors, such as flexible, tattoo-like sensors based on microfluidics, are also promising for non-invasive, haemodynamic, continuous monitoring. However, all these emerging sensors still require extensive clinical validation 33 .
Figure 1 summarizes common smart wearable devices, their embedded sensors and their applications in cardiovascular care. Table 1 lists common wearable products on the market, the published studies on these products and their regulatory status.
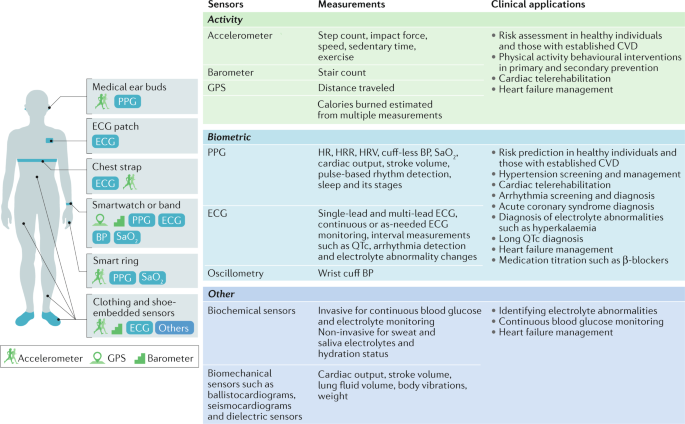
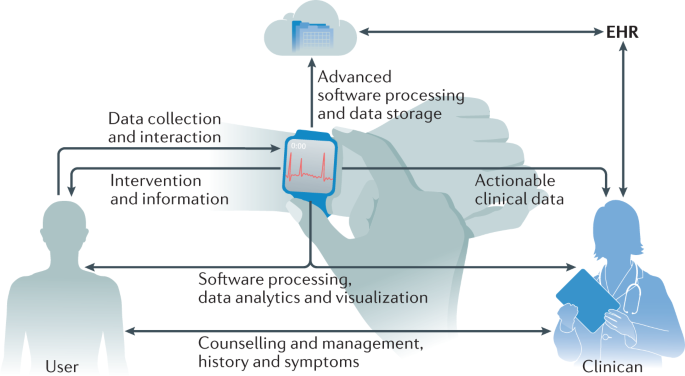
A practical ABCD guide for clinicians
As consumers continue to push the use of wearable data in clinical care and as health-care leaders and policymakers sift through all the challenges mentioned above, clinicians, in the meantime, would benefit from a pragmatic guide to evaluate commercial wearables and integrate them into their clinical practice. We recommend a simple ABCD guide that clinicians can utilize in their daily practice (Table 4). Through this guide, clinicians can assess the device’s accuracy, clinical utility and validity, regulatory approval status, price, and any existing best-practice guidelines. Clinicians can then determine whether the device benefits their patients and clinical practice in terms of quality of care, efficiency, convenience and cost-effectiveness. After the device is deemed to have reasonable accuracy and clinical utility, clinical workflow integration needs to be established. This workflow includes integration with electronic health records, establishing logistics for patient onboarding and staff education, setting parameter alarms and expectations for the frequency of data review, and the development of reimbursement or payment structures. Finally, clinicians must institute data rights and governance procedures through state-of-the-art data user agreements and privacy policies that maintain patient confidentiality and trust. This simple guide can potentially accelerate wearable integration in cardiovascular practice and usher a new era of connected remote patient care.
Table 4 A clinician’s ABCD guide to wearable device use in clinical practice
Conclusions
A new age of consumer-driven health has arrived, with great future benefits in cardiovascular disease prevention, diagnosis and management. Currently, several challenges hinder the widespread use of wearable technologies in clinical practice. As sensor and computing technologies continue to evolve, wearables will acquire more complex functions and become an integral part of our cardiovascular practice armamentarium. These devices must be regulated through comprehensive evaluation frameworks and adequate regulatory oversight policies to ensure safety and efficacy. Moreover, a practical ABCD clinician’s guide can facilitate the integration of these devices into the clinical workplace. As COVID-19 has launched us at rocket speed into a new era of remote and decentralized patient care, this is a golden opportunity to shake off our scepticism and embrace wearable technologies in our clinical practices for the benefit of our patients.
References
- Sana, F. et al. Wearable devices for ambulatory cardiac monitoring. J. Am. Coll. Cardiol.75, 1582–1592 (2020). ArticlePubMedPubMed CentralGoogle Scholar
- Polaris Market Research. Healthcare analytics market share, size, trends, industry analysis report, 2021–2028. Polaris Market Researchhttps://www.polarismarketresearch.com/industry-analysis/wearable-medical-devices-market (2020).
- Linden, A. & Fenn, J. Understanding Gartner’s Hype Cycles. http://www.ask-force.org/web/Discourse/Linden-HypeCycle-2003.pdf (2003).
- Varma, N. et al. HRS/EHRA/APHRS/LAHRS/ACC/AHA worldwide practice update for telehealth and arrhythmia monitoring during and after a Pandemic. Circ. Arrhythm. Electrophysiol.13, 1048–1059 (2020). Google Scholar
- Blond, K., Brinkløv, C. F., Ried-Larsen, M., Crippa, A. & Grøntved, A. Association of high amounts of physical activity with mortality risk: a systematic review and meta-analysis. Br. J. Sports Med.54, 1195–1201 (2020). ArticlePubMedGoogle Scholar
- American Heart Association. Life’s Simple 7. AHAhttps://www.heart.org/en/professional/workplace-health/lifes-simple-7 (2018).
- Yang, C. C. & Hsu, Y. L. A review of accelerometry-based wearable motion detectors for physical activity monitoring. Sensors10, 7772–7788 (2010). ArticlePubMedPubMed CentralGoogle Scholar
- Troiano, R. P., McClain, J. J., Brychta, R. J. & Chen, K. Y. Evolution of accelerometer methods for physical activity research. Br. J. Sports Med.48, 1019–1023 (2014). ArticlePubMedGoogle Scholar
- GPS.gov. How GPS works. GPS.govhttps://www.gps.gov/multimedia/poster/ (2020).
- Bolanakis, D. E. MEMS barometers and barometric altimeters in industrial, medical, aerospace, and consumer applications. IEEE Instrum. Meas. Mag.20, 30–55 (2017). ArticleGoogle Scholar
- Muralidharan, K., Khan, A. J., Misra, A., Balan, R. K. & Agarwal, S. Barometric phone sensors: more hype than hope! Proc. Workshop Mob. Comput. Syst. Appl.14, 1–6 (2014). Google Scholar
- Zhang, D., Wang, W. & Li, F. Association between resting heart rate and coronary artery disease, stroke, sudden death and noncardiovascular diseases: a meta-analysis. Can. Med. Assoc. J.188, E384–E392 (2016). ArticleGoogle Scholar
- Fox, K. et al. Heart rate as a prognostic risk factor in patients with coronary artery disease and left-ventricular systolic dysfunction (BEAUTIFUL): a subgroup analysis of a randomised controlled trial. Lancet372, 817–821 (2008). ArticlePubMedGoogle Scholar
- Sydó, N. et al. Prognostic performance of heart rate recovery on an exercise test in a primary prevention population. J. Am. Heart Assoc.7, e008143 (2018). ArticlePubMedPubMed CentralGoogle Scholar
- Singh, N. et al. Heart rate variability: an old metric with new meaning in the era of using mHealth technologies for Health and Exercise Training Guidance. Part Two: Prognosis and Training. Arrhythmia Electrophysiol. Rev.7, 247–255 (2018). Google Scholar
- Samol, A. et al. Single-lead ECG recordings including Einthoven and Wilson leads by a smartwatch: a new era of patient directed early ECG differential diagnosis of cardiac diseases? Sensors19, 4377 (2019). ArticlePubMed CentralGoogle Scholar
- Cobos Gil, M. Á. Standard and precordial leads obtained with an apple watch. Ann. Intern. Med.172, 436 (2020). ArticlePubMedGoogle Scholar
- Kamišalić, A., Fister, I., Turkanović, M. & Karakatič, S. Sensors and functionalities of non-invasive wrist-wearable devices: a review. Sensors18, 1714 (2018). ArticlePubMed CentralGoogle Scholar
- Perez, M. V. et al. Large-scale assessment of a smartwatch to identify atrial fibrillation. N. Engl. J. Med.381, 1909–1917 (2019). ArticlePubMedPubMed CentralGoogle Scholar
- Dagher, L., Shi, H., Zhao, Y. & Marrouche, N. F. Wearables in cardiology: here to stay. Heart Rhythm17, 889–895 (2020). ArticlePubMedGoogle Scholar
- Bent, B., Goldstein, B. A., Kibbe, W. A. & Dunn, J. P. Investigating sources of inaccuracy in wearable optical heart rate sensors. NPJ Digit. Med.3, 18 (2020). ArticlePubMedPubMed CentralGoogle Scholar
- Nelson, B. W. & Allen, N. B. Accuracy of consumer wearable heart rate measurement during an ecologically valid 24-hour period: intraindividual validation study. JMIR mHealth uHealth7, e10828 (2019). ArticlePubMedPubMed CentralGoogle Scholar
- Etiwy, M. et al. Accuracy of wearable heart rate monitors in cardiac rehabilitation. Cardiovasc. Diagn. Ther.9, 262–271 (2019). ArticlePubMedPubMed CentralGoogle Scholar
- Whelton, P. K. et al. 2017 ACC/AHA/AAPA/ABC/ACPM/AGS/APhA/ASH/ASPC/NMA/PCNA Guideline for the prevention, detection, evaluation, and management of high blood pressure in adults: executive summary: a report of the American College of Cardiology/American Heart Association Task Force on Clinical Practice Guidelines. Circulation138, e426–e483 (2018). PubMedGoogle Scholar
- Kario, K. et al. The first study comparing a wearable watch-type blood pressure monitor with a conventional ambulatory blood pressure monitor on in-office and out-of-office settings. J. Clin. Hypertens.22, 135–141 (2020). ArticleGoogle Scholar
- Zweiker, R., Schumacher, M., Fruhwald, F. M., Watzinger, N. & Klein, W. Comparison of wrist blood pressure measurement with conventional sphygmomanometry at a cardiology outpatient clinic. J. Hypertens.18, 1013–1018 (2000). ArticleCASPubMedGoogle Scholar
- Bard, D. M., Joseph, J. I. & van Helmond, N. Cuff-less methods for blood pressure telemonitoring. Front. Cardiovasc. Med.6, 40 (2019). ArticlePubMedPubMed CentralGoogle Scholar
- Islam, S. M. S. et al. Validation and acceptability of a cuffless wrist-worn wearable blood pressure monitoring device among users and health care professionals: mixed methods study. JMIR mHealth uHealth7, e14706 (2019). ArticlePubMedPubMed CentralGoogle Scholar
- McCombie, D. B., Reisner, A. T. & Asada, H. H. Adaptive blood pressure estimation from wearable PPG sensors using peripheral artery pulse wave velocity measurements and multi-channel blind identification of local arterial dynamics. Conf. Proc. IEEE Eng. Med. Biol. Soc.2006, 3521–3524 (2006). ArticlePubMedGoogle Scholar
- Kim, J., Campbell, A. S., de Ávila, B. E.-F. & Wang, J. Wearable biosensors for healthcare monitoring. Nat. Biotechnol.37, 389–406 (2019). ArticleCASPubMedPubMed CentralGoogle Scholar
- Bailey, T. S. Clinical implications of accuracy measurements of continuous glucose sensors. Diabetes Technol. Ther.19 (Suppl. 2), S51–S54 (2017). ArticlePubMedGoogle Scholar
- Seshadri, D. R. et al. Wearable sensors for monitoring the physiological and biochemical profile of the athlete. NPJ Digit. Med.2, 72 (2019). ArticlePubMedPubMed CentralGoogle Scholar
- Digiglio, P., Li, R., Wang, W. & Pan, T. Microflotronic arterial tonometry for continuous wearable non-invasive hemodynamic monitoring. Ann. Biomed. Eng.42, 2278–2288 (2014). ArticlePubMedGoogle Scholar
- Borgundvaag, E. & Janssen, I. Objectively measured physical activity and mortality risk among American adults. Am. J. Prev. Med.52, e25–e31 (2017). ArticlePubMedGoogle Scholar
- Dohrn, I. M., Sjöström, M., Kwak, L., Oja, P. & Hagströmer, M. Accelerometer-measured sedentary time and physical activity — A 15 year follow-up of mortality in a Swedish population-based cohort. J. Sci. Med. Sport.21, 702–707 (2018). ArticlePubMedGoogle Scholar
- Klenk, J. et al. Objectively measured walking duration and sedentary behaviour and four-year mortality in older people. PLoS ONE11, e0153779 (2016). ArticlePubMedPubMed CentralCASGoogle Scholar
- LaMonte, M. J. et al. Accelerometer-measured physical activity and mortality in women aged 63 to 99. J. Am. Geriatr. Soc.66, 886–894 (2018). ArticlePubMedGoogle Scholar
- Lee, I. M. et al. Accelerometer-measured physical activity and sedentary behavior in relation to all-cause mortality: the women’s health study. Circulation137, 203–205 (2018). ArticlePubMedGoogle Scholar
- Lee, I.-M. et al. Association of step volume and intensity with all-cause mortality in older women. JAMA Intern. Med.179, 1105 (2019). ArticlePubMedPubMed CentralGoogle Scholar
- Martin, S. S. et al. mActive: a randomized clinical trial of an automated mHealth intervention for physical activity promotion. J. Am. Heart Assoc.4, e002239 (2015). ArticlePubMedPubMed CentralGoogle Scholar
- Patel, M. S. et al. Effect of a game-based intervention designed to enhance social incentives to increase physical activity among families. JAMA Intern. Med.177, 1586 (2017). ArticlePubMedPubMed CentralGoogle Scholar
- Gremaud, A. L. et al. Gamifying accelerometer use increases physical activity levels of sedentary office workers. J. Am. Heart Assoc.7, e007735 (2018). ArticlePubMedPubMed CentralGoogle Scholar
- Adams, M. A. et al. Adaptive goal setting and financial incentives: a 2 × 2 factorial randomized controlled trial to increase adults’ physical activity. BMC Public Health17, 286 (2017). ArticlePubMedPubMed CentralGoogle Scholar
- Finkelstein, E. A. et al. Effectiveness of activity trackers with and without incentives to increase physical activity (TRIPPA): a randomised controlled trial. Lancet Diabetes Endocrinol.4, 983–995 (2016). ArticlePubMedGoogle Scholar
- Wang, J. B. et al. Wearable sensor/device (Fitbit One) and SMS text-messaging prompts to increase physical activity in overweight and obese adults: a randomized controlled trial. Telemed. e-Health21, 782–792 (2015). ArticleGoogle Scholar
- Quer, G., Gouda, P., Galarnyk, M., Topol, E. J. & Steinhubl, S. R. Inter- and intraindividual variability in daily resting heart rate and its associations with age, sex, sleep, BMI, and time of year: retrospective, longitudinal cohort study of 92,457 adults. PLoS ONE15, e0227709 (2020). ArticleCASPubMedPubMed CentralGoogle Scholar
- American Heart Association. Know your target heart rates for exercise, losing weight and health. AHAhttps://www.heart.org/en/healthy-living/fitness/fitness-basics/target-heart-rates (2015).
- Kuwabara, M., Harada, K., Hishiki, Y. & Kario, K. Validation of two watch-type wearable blood pressure monitors according to the ANSI/AAMI/ISO81060-2:2013 guidelines: Omron HEM-6410T-ZM and HEM-6410T-ZL. J. Clin. Hypertens.21, 853–858 (2019). ArticleGoogle Scholar
- Kirchhof, P. et al. 2016 ESC guidelines for the management of atrial fibrillation developed in collaboration with EACTS. Eur. Heart J.37, 2893–2962 (2016). ArticlePubMedGoogle Scholar
- Steinhubl, S. R. et al. Effect of a home-based wearable continuous ECG monitoring patch on detection of undiagnosed atrial fibrillation. JAMA320, 146 (2018). ArticlePubMedPubMed CentralGoogle Scholar
- Tison, G. H. et al. Passive detection of atrial fibrillation using a commercially available smartwatch. JAMA Cardiol.3, 409 (2018). ArticlePubMedPubMed CentralGoogle Scholar
- Fan, Y. Y. et al. Diagnostic performance of a smart device with photoplethysmography technology for atrial fibrillation detection: pilot study (Pre-mAFA II registry). JMIR Mhealth Uhealth.7, e11437 (2019). ArticlePubMedPubMed CentralGoogle Scholar
- Bumgarner, J. M. et al. Smartwatch algorithm for automated detection of atrial fibrillation. J. Am. Coll. Cardiol.71, 2381–2388 (2018). ArticlePubMedGoogle Scholar
- US National Library of Medicine. ClinicalTrials.govhttps://clinicaltrials.gov/ct2/show/NCT04276441 (2020).
- Barrett, P. M. et al. Comparison of 24-hour Holter monitoring with 14-day novel adhesive patch electrocardiographic monitoring. Am. J. Med.127, 95.e11–95.e17 (2014). ArticleGoogle Scholar
- Turakhia, M. P. et al. Diagnostic utility of a novel leadless arrhythmia monitoring device. Am. J. Cardiol.112, 520–524 (2013). ArticlePubMedGoogle Scholar
- Hannun, A. Y. et al. Cardiologist-level arrhythmia detection and classification in ambulatory electrocardiograms using a deep neural network. Nat. Med.25, 65–69 (2019). ArticleCASPubMedPubMed CentralGoogle Scholar
- Reed, M. J. et al. Multi-centre randomised controlled trial of a smartphone-based event recorder alongside standard care versus standard care for patients presenting to the emergency department with palpitations and pre-syncope: the IPED (Investigation of Palpitations in the ED) study. EClinicalMedicine8, 37–46 (2019). ArticlePubMedPubMed CentralGoogle Scholar
- Ballinger, B. et al. DeepHeart: semi-supervised sequence learning for cardiovascular risk prediction. AAAI Conf. Artif. Intell.32, 2079–2086 (2018). Google Scholar
- Attia, Z. I. et al. Screening for cardiac contractile dysfunction using an artificial intelligence–enabled electrocardiogram. Nat. Med.25, 70–74 (2019). ArticleCASPubMedGoogle Scholar
- Sopic, D., Aminifar, A., Aminifar, A. & Atienza, D. Real-time event-driven classification technique for early detection and prevention of myocardial infarction on wearable systems. IEEE Trans. Biomed. Circuits Syst.12, 982–992 (2018). ArticleGoogle Scholar
- Werhahn, S. M. et al. Designing meaningful outcome parameters using mobile technology: a new mobile application for telemonitoring of patients with heart failure. ESC Heart Fail.6, 516–525 (2019). ArticlePubMedPubMed CentralGoogle Scholar
- Sherazi, S. et al. Prognostic significance of heart rate variability among patients treated with cardiac resynchronization therapy. JACC Clin. Electrophysiol.1, 74–80 (2015). ArticlePubMedGoogle Scholar
- Gensini, G. F., Alderighi, C., Rasoini, R., Mazzanti, M. & Casolo, G. Value of telemonitoring and telemedicine in heart failure management. Card. Fail. Rev.3, 116–121 (2017). ArticlePubMedPubMed CentralGoogle Scholar
- Abraham, W. T. et al. Wireless pulmonary artery haemodynamic monitoring in chronic heart failure: a randomised controlled trial. Lancet377, 658–666 (2011). ArticlePubMedGoogle Scholar
- Koehler, F. et al. Efficacy of telemedical interventional management in patients with heart failure (TIM-HF2): a randomised, controlled, parallel-group, unmasked trial. Lancet392, 1047–1057 (2018). ArticlePubMedGoogle Scholar
- Dendale, P. et al. Effect of a telemonitoring-facilitated collaboration between general practitioner and heart failure clinic on mortality and rehospitalization rates in severe heart failure: the TEMA-HF 1 (telemonitoring in the management of heart failure) study. Eur. J. Heart Fail.14, 333–340 (2012). ArticlePubMedGoogle Scholar
- Cleland, J. G. F., Louis, A. A., Rigby, A. S., Janssens, U. & Balk, A. H. M. M. Noninvasive home telemonitoring for patients with heart failure at high risk of recurrent admission and death: the trans-European network-home-care management system (TEN-HMS) study. J. Am. Coll. Cardiol.45, 1654–1664 (2005). ArticlePubMedGoogle Scholar
- Koehler, F. et al. Impact of remote telemedical management on mortality and hospitalizations in ambulatory patients with chronic heart failure: the telemedical interventional monitoring in heart failure study. Circulation123, 1873–1880 (2011). ArticlePubMedGoogle Scholar
- Ong, M. K. et al. Effectiveness of remote patient monitoring after discharge of hospitalized patients with heart failure the better effectiveness after transition-heart failure (BEAT-HF) randomized clinical trial. JAMA Intern. Med.176, 310–318 (2016). ArticlePubMedPubMed CentralGoogle Scholar
- Stavrakis, S. et al. Intermittent vs. continuous anticoagulation therapy in patients with atrial fibrillation (iCARE-Af): a randomized pilot study. J. Interv. Card. Electrophysiol.48, 51–60 (2017). ArticlePubMedGoogle Scholar
- Zado, E. S. et al. “As Needed” nonvitamin K antagonist oral anticoagulants for infrequent atrial fibrillation episodes following atrial fibrillation ablation guided by diligent pulse monitoring: a feasibility study. J. Cardiovasc. Electrophysiol.30, 631–638 (2019). ArticlePubMedGoogle Scholar
- Passman, R. et al. Targeted anticoagulation for atrial fibrillation guided by continuous rhythm assessment with an insertable cardiac monitor: the Rhythm Evaluation for Anticoagulation with Continuous Monitoring (REACT.COM) Pilot Study. J. Cardiovasc. Electrophysiol.27, 264–270 (2016). ArticlePubMedGoogle Scholar
- Steinhaus, D. A., Zimetbaum, P. J., Passman, R. S., Leong-Sit, P. & Reynolds, M. R. Cost effectiveness of implantable cardiac monitor-guided intermittent anticoagulation for atrial fibrillation: an analysis of the REACT.COM pilot study. J. Cardiovasc. Electrophysiol.27, 1304–1311 (2016). ArticlePubMedPubMed CentralGoogle Scholar
- Elshazly, M. B. et al. Exercise ventricular rates, cardiopulmonary exercise performance, and mortality in patients with heart failure with atrial fibrillation. Circ. Heart Fail.14, e007451 (2021). ArticlePubMedGoogle Scholar
- Marvel, F. A., Spaulding, E. M., Lee, M., Yang, W. & Martin, S. S. Abstract 26: The Corrie Myocardial infarction, COmbined-device, Recovery Enhancement (MiCORE) study: 30-day readmission rates and cost-effectiveness of a novel digital health intervention for acute myocardial infarction patients. Circ. Cardiovasc. Qual. Outcomes12, (2019).
- McKeown, L.A. Digital tools show promise for helping STEMI and NSTEMI patients avoid readmission. TCTMDhttps://www.tctmd.com/news/digital-tools-show-promise-helping-stemi-and-nstemi-patients-avoid-readmission (2019).
- Castelletti, S. et al. A wearable remote monitoring system for the identification of subjects with a prolonged QT interval or at risk for drug-induced long QT syndrome. Int. J. Cardiol.266, 89–94 (2018). ArticlePubMedGoogle Scholar
- Schram, M. et al. Prediction of the heart rate corrected QT interval (QTc) from a novel, multilead smartphone-enabled ECG using a deep neural network. J. Am. Coll. Cardiol.73, 368 (2019). ArticleGoogle Scholar
- Rosano, G. M. C. et al. Expert consensus document on the management of hyperkalaemia in patients with cardiovascular disease treated with renin angiotensin aldosterone system inhibitors: coordinated by the Working Group on Cardiovascular Pharmacotherapy of the European Society of Cardiology. Eur. Hear. J. Cardiovasc. Pharmacother.4, 180–188 (2018). ArticleGoogle Scholar
- Rafique, Z., Chouihed, T., Mebazaa, A. & Frank Peacock, W. Current treatment and unmet needs of hyperkalaemia in the emergency department. Eur. Hear. J. Suppl.21 (Suppl. A), A12–A19 (2019). ArticleCASGoogle Scholar
- Galloway, C. D. et al. Development and validation of a deep-learning model to screen for hyperkalemia from the electrocardiogram. JAMA Cardiol.4, 428 (2019). ArticlePubMedPubMed CentralGoogle Scholar
- Galloway, C. D. et al. Non-invasive detection of hyperkalemia with a smartphone electrocardiogram and artificial intelligence. J. Am. Coll. Cardiol.71, A272 (2018). ArticleGoogle Scholar
- Anderson, L. et al. Home-based versus centre-based cardiac rehabilitation. Cochrane Database Syst. Rev.6, CD007130 (2017). PubMedGoogle Scholar
- Maddison, R. et al. Effects and costs of real-time cardiac telerehabilitation: randomised controlled non-inferiority trial. Heart105, 122–129 (2019). ArticlePubMedGoogle Scholar
- Hannan, A. L. et al. Impact of wearable physical activity monitoring devices with exercise prescription or advice in the maintenance phase of cardiac rehabilitation: systematic review and meta-analysis. BMC Sports Sci. Med. Rehabil.11, 14 (2019). ArticlePubMedPubMed CentralGoogle Scholar
- Normahani, P. et al. Wearable Sensor Technology Efficacy in Peripheral Vascular Disease (wSTEP). Ann. Surg.268, 1113–1118 (2018). ArticlePubMedGoogle Scholar
- Gardner, A. W., Parker, D. E., Montgomery, P. S. & Blevins, S. M. Step-monitored home exercise improves ambulation, vascular function, and inflammation in symptomatic patients with peripheral artery disease: a randomized controlled trial. J. Am. Heart Assoc.3, e001107 (2014). ArticlePubMedPubMed CentralGoogle Scholar
- Gardner, A. W., Parker, D. E., Montgomery, P. S., Scott, K. J. & Blevins, S. M. Efficacy of quantified home-based exercise and supervised exercise in patients with intermittent claudication: a randomized controlled trial. Circulation123, 491–498 (2011). ArticlePubMedPubMed CentralGoogle Scholar
- McDermott, M. M. et al. Effect of a home-based exercise intervention of wearable technology and telephone coaching on walking performance in peripheral artery disease. JAMA319, 1665 (2018). ArticlePubMedPubMed CentralGoogle Scholar
- Chan, C. et al. The role of wearable technologies and telemonitoring in managing vascular disease. Vasc. Endovasc. Rev.https://doi.org/10.15420/ver.2019.11 (2020). ArticleGoogle Scholar
- Shcherbina, A. et al. Accuracy in wrist-worn, sensor-based measurements of heart rate and energy expenditure in a diverse cohort. J. Pers. Med.7, 3 (2017). ArticlePubMed CentralGoogle Scholar
- Vetrovsky, T. et al. Validity of six consumer-level activity monitors for measuring steps in patients with chronic heart failure. PLoS ONE14, e0222569 (2019). ArticleCASPubMedPubMed CentralGoogle Scholar
- Herkert, C., Kraal, J. J., van Loon, E. M. A., van Hooff, M. & Kemps, H. M. C. Usefulness of modern activity trackers for monitoring exercise behavior in chronic cardiac patients: validation study. JMIR mHealth uHealth7, e15045 (2019). ArticlePubMedPubMed CentralGoogle Scholar
- Coravos, A. et al. Modernizing and designing evaluation frameworks for connected sensor technologies in medicine. NPJ Digit. Med.3, 37 (2020). ArticlePubMedPubMed CentralGoogle Scholar
- US Food and Drug Administration. Classify your medical device. FDAhttp://www.fda.gov/MedicalDevices/DeviceRegulationandGuidance/Overview/ClassifyYourDevice/ (2016).
- Matheny, M. E., Whicher, D. & Thadaney Israni, S. Artificial intelligence in health care: a report from the national academy of medicine. JAMA323, 509–510 (2020). ArticlePubMedGoogle Scholar
- Haibe-Kains, B. et al. Transparency and reproducibility in artificial intelligence. Nature586, E14–E16 (2020). ArticleCASPubMedPubMed CentralGoogle Scholar
- Matheny, M., Israni, S. T., Ahmed, M., Whicher, D. & Edu, N. Artificial intelligence in health care: the hope, the hype, the promise, the peril (National Academy of Medicine, 2019).
- US Food and Drug Administration. Digital health software precertification (Pre-Cert) program: participate in 2019 test plan. FDAhttps://www.fda.gov/medical-devices/digital-health-software-precertification-pre-cert-program/digital-health-software-precertification-pre-cert-program-participate-2019-test-plan (2019).
- Marcus, G. M. The apple watch can detect atrial fibrillation: so what now? Nat. Rev. Cardiol.17, 135–136 (2020). ArticleCASPubMedGoogle Scholar
- Major, S., Sawan, L., Vognsen, J. & Jabre, M. COVID-19 pandemic prompts the development of a Web-OSCE using Zoom teleconferencing to resume medical students’ clinical skills training at Weill Cornell Medicine-Qatar. BMJ Simul. Technol. Enhanc. Learn.6, 376–377 (2020). ArticlePubMed CentralGoogle Scholar
- Yardley, L., Choudhury, T., Patrick, K. & Michie, S. Current issues and future directions for research into digital behavior change interventions. Am. J. Prev. Med.51, 814–815 (2016). ArticlePubMedGoogle Scholar
- Hekler, E. B. et al. Advancing models and theories for digital behavior change interventions. Am. J. Prev. Med.51, 825–832 (2016). ArticlePubMedPubMed CentralGoogle Scholar
- Zhou, M., Fukuoka, Y., Goldberg, K., Vittinghoff, E. & Aswani, A. Applying machine learning to predict future adherence to physical activity programs. BMC Med. Inform. Decis. Mak.19, 169 (2019). ArticlePubMedPubMed CentralGoogle Scholar
- Fitbit. Fitbit launches Fitbit Care, a powerful new enterprise health platform for wellness and prevention and disease management. Fitbithttps://investor.fitbit.com/press/press-releases/press-release-details/2018/Fitbit-Launches-Fitbit-Care-A-Powerful-New-Enterprise-Health-Platform-for-Wellness-and-Prevention-and-Disease-Management/default.aspx (2018).
- Vogels, E. A. About one-in-five Americans use a smart watch or fitness tracker. Pew Research Centerhttps://www.pewresearch.org/fact-tank/2020/01/09/about-one-in-five-americans-use-a-smart-watch-or-fitness-tracker (2020).
- Chia, G. L. C., Anderson, A. & McLean, L. A. Behavior change techniques incorporated in fitness trackers: content analysis. JMIR mHealth uHealth7, e12768 (2019). ArticlePubMedPubMed CentralGoogle Scholar
- Yang, W. E. et al. Strategies for the successful implementation of a novel iPhone Loaner System (iShare) in mHealth interventions: prospective study. JMIR mHealth uHealth7, e16391 (2019). ArticlePubMedPubMed CentralGoogle Scholar
- Cohen, I. G. & Mello, M. M. Big data, big tech, and protecting patient privacy. JAMA322, 1141 (2019). ArticlePubMedGoogle Scholar
- Hasselgren, A., Kralevska, K., Gligoroski, D., Pedersen, S. A. & Faxvaag, A. Blockchain in healthcare and health sciences—a scoping review. Int. J. Med. Inf.134, 104040 (2020). ArticleGoogle Scholar
- Sarpatwari, A. & Choudhry, N. K. Recalibrating privacy protections to promote patient engagement. N. Engl. J. Med.377, 1509–1511 (2017). ArticlePubMedGoogle Scholar
- Slotwiner, D. J. et al. Transparent sharing of digital health data: a call to action. Heart Rhythm.16, e95–e106 (2019). ArticlePubMedGoogle Scholar
- Rosenbaum, L. Google Health exec defends controversial partnership with Ascension: ‘We’re super proud of it’. https://www.forbes.com/sites/leahrosenbaum/2020/01/14/google-health-exec-defends-controversial-partnership-with-ascension-were-super-proud-of-it (2020).
- mTelehealth. CMS guidance for remote patient monitoring (RPM) during COVID-19 (CPT Code 99453). mTelehealthhttps://mtelehealth.com/cms-guidance-for-remote-patient-monitoring-rpm-during-covid-19-cpt-code-99453/ (2020).
Acknowledgements
The authors express their appreciation to S. Steinhubl (Director, Digital Medicine, Scripps Research Translation Institute, San Diego, CA, USA) and M. Wahba (CEO, EMBER Medical, USA) for providing guidance and comments on the manuscript. S.S.M. has received funding from the Aetna Foundation, AHA, CASCADE FH, David and June Trone Family Foundation, the Pollin Digital Innovation fund and NIH (P01 HL108800). M.P.T. has received funding from the AHA.
Author information
- These authors contributed equally: Karim Bayoumy, Mohammed Gaber.
Authors and Affiliations
- Department of Medicine, NewYork-Presbyterian Brooklyn Methodist Hospital, Brooklyn, NY, USA Karim Bayoumy
- Department of Oncology, National Center for Cancer Care and Research, Hamad Medical Corporation, Doha, Qatar Mohammed Gaber
- Department of Medical Education, Weill Cornell Medicine, Doha, Qatar Abdallah Elshafeey, Omar Mhaimeed & Mohamed B. Elshazly
- Department of Cardiovascular Medicine, University of California Irvine, Irvine, CA, USA Elizabeth H. Dineen
- Johns Hopkins Ciccarone Center for the Prevention of Cardiovascular Disease, Baltimore, MD, USA Francoise A. Marvel, Seth S. Martin & Mohamed B. Elshazly
- Scripps Research Translational Institute and Division of Cardiovascular Diseases, Scripps Clinic, La Jolla, CA, USA Evan D. Muse
- Center for Digital Health, Stanford University, Stanford, CA, USA Mintu P. Turakhia
- VA Palo Alto Health Care System, Palo Alto, CA, USA Mintu P. Turakhia
- Department of Cardiovascular Medicine, Heart and Vascular Institute, Cleveland Clinic, Cleveland, OH, USA Khaldoun G. Tarakji
- Department of Medicine, Weill Cornell Medicine, New York, NY, USA Mohamed B. Elshazly
- Karim Bayoumy